Substantially Reduce Observability Costs with Asserts Data Distiller
Observability produces a lot of data which consumes compute and storage resources with their associated costs. Asserts Data Distiller observes everything only retaining what matters, significantly reducing costs.

The crux of Observability is gaining visibility into running applications, lifting the lid on the black box and peering inside at the inner workings. The more metrics, logs and traces collected the better the visibility, Murphy’s Law clearly states that the item of information needed at a critical time is the one that was not collected. The paradox is that the vast majority of the data collected is only pertinent for a relatively short time and can be discarded once its usefulness has expired.
Observability platform vendors play on our insecurity that we might miss an important data point and therefore encourage us to collect ever increasing amounts of data, keeping it for as long as possible. No surprise that they play on our paranoia, their pricing structures are directly tied to the volume of data; more data equals more money. For many organisations the observability platform bill runs a close second to the cloud platform bill. Perhaps there is a more cost efficient way to achieve maximum observability at minimum cost? Organisations should not compromise visibility in fear of the bill.
Observe Everything Retain Only What Matters
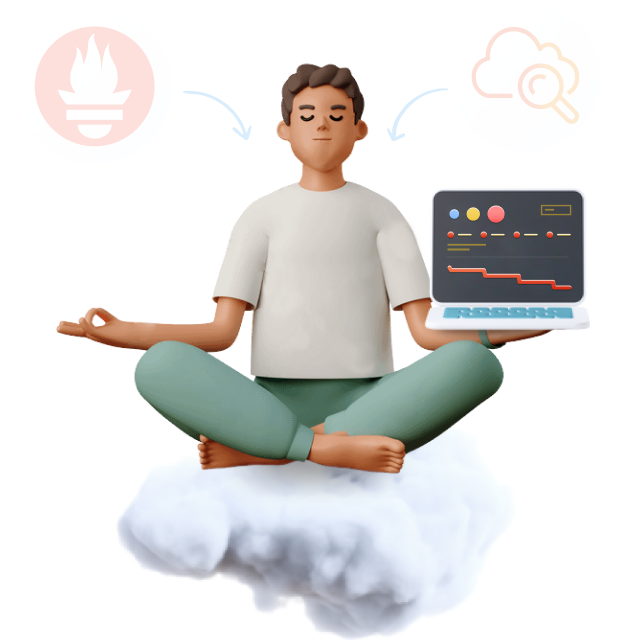
The primary goal of observability is to enable an organisation to know that their transactional applications are serving requests in a prompt and error free manner. If an application should fail to meet its Service Level Objectives, collected observability data should provide sufficient insights to enable DevOps and SRE teams to identify possible solutions to restore service levels. Only a small subset of this data is required for long term reporting, for example, knowing system CPU usage on a VM that was deprovisioned six months ago is a total waste of space.
Clearly there are essentially two tiers required for efficient management of observability data: high density short term and low density long term storage.
Asserts Data Distiller™ automates data efficiency for your observability data. The Prometheus exporters and client libraries capture a richer set of metrics than their commercial equivalents, ensuring maximum visibility. Asserts Data Distiller™ queries the short term metric store(s), normalises the metric data, calculates baselines and persists it in its own Prometheus compatible long term metric store. The normalised long term data is 10 - 20 times smaller in storage and compute requirements than the high cardinality metric data making it cost effective for long term reporting.
Traces are the greatest overhead both in terms of processing compute and storage requirements. Commercial observability tools offer head and/or tail based sampling in an attempt to provide you with some control over which traces are captured and/or retained and therefore make an effort to constrain the costs. Open Telemetry only offers head based sampling, which is a blunt instrument with a very high possibility of missing some useful traces; remember Murphy’s Law. Tail based sampling is better because it can apply intelligent algorithms across all trace data. However, there is extra cost involved: the observability provider will charge for the extra trace data processed and the cloud provider will charge for the extra data egress.
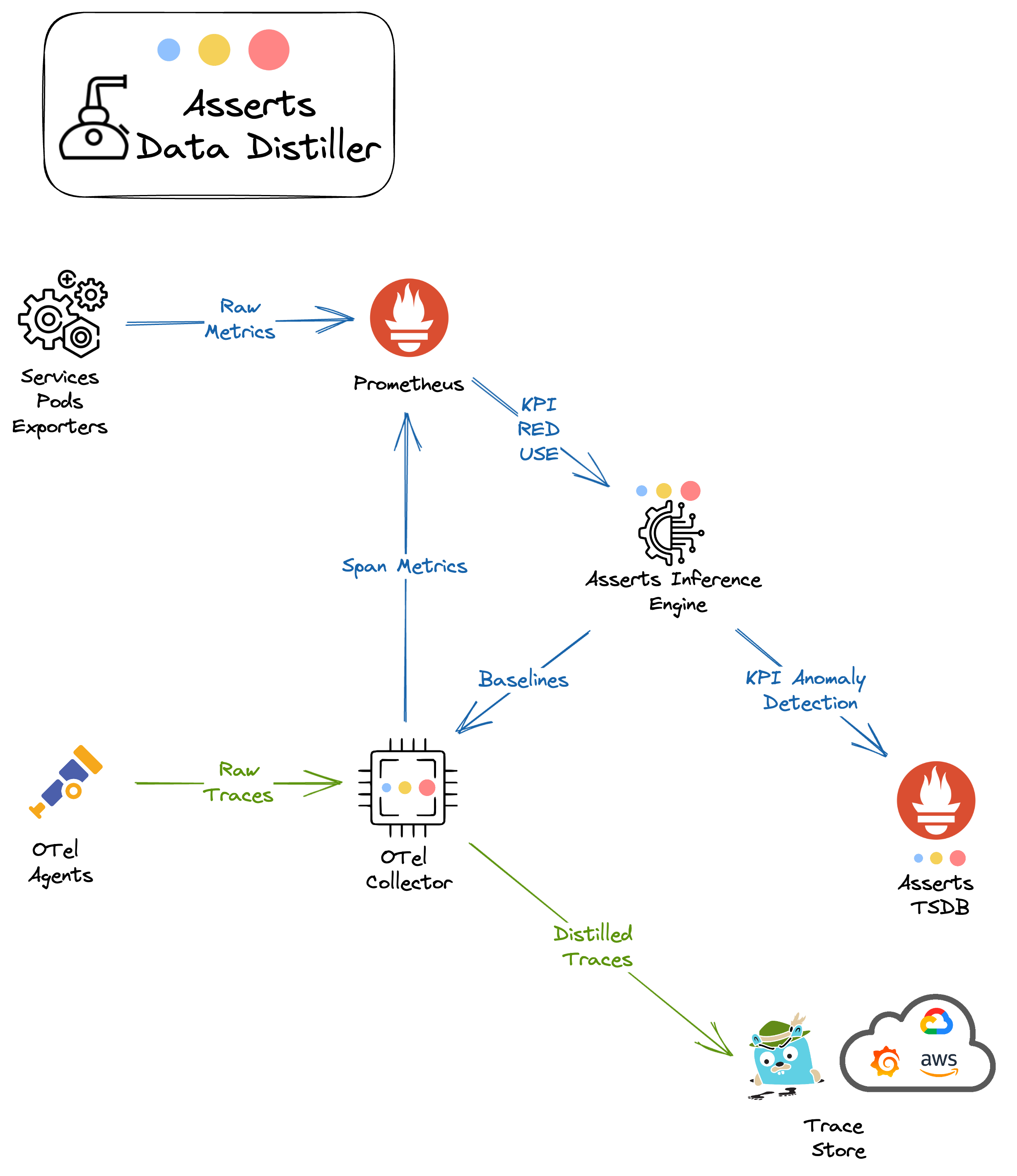
Asserts Data Distiller™ uses the best of both approaches for trace data. It is a processor that plugs into the Open Telemetry collector. All received spans are processed and directly converted into RED and relationship metrics which go directly into the high resolution metric data store. This information is used by Asserts to generate baselines and the Entity Graph. The calculated baseline information is queried by the distiller from the Asserts data store and used to determine if a trace is slow. Only slow traces, those with the error flag set and periodic traces are forwarded to the tracing store e.g. Jaeger. This intelligent approach to trace sampling considerably reduces trace processing and storage requirements while guaranteeing that important information is not dropped.
Maximum Observability Minimum Cost
Observability is mandatory but it does not have to cost so much. Asserts Data Distiller™ provides maximum observability at high resolution while efficiently managing data processing and storage requirements thus reducing costs.
An application with a Service Level Objective of 99% of requests being prompt and error free. If the application stays just within its SLO, then only 1% of the request traces will be captured; just the slow and erroneous ones. If traces were randomly sampled at just 1%, too many useful traces would be dropped and the collected traces would not provide sufficient information to troubleshoot any incidents. Asserts Data Distiller™ intelligent sampling provides complete visibility with maximum efficiency, significantly reducing the costs associated with tracing and long term metric storage.
Take control of your observability data and start using Asserts today free forever. Install the Helm chart on your own Kubernetes cluster and start saving on your observability costs today.